Multi-view Mask/Depth Supervision (ShapeNet)
|
|
Single-view Mask Supervision (PASCAL VOC)
|
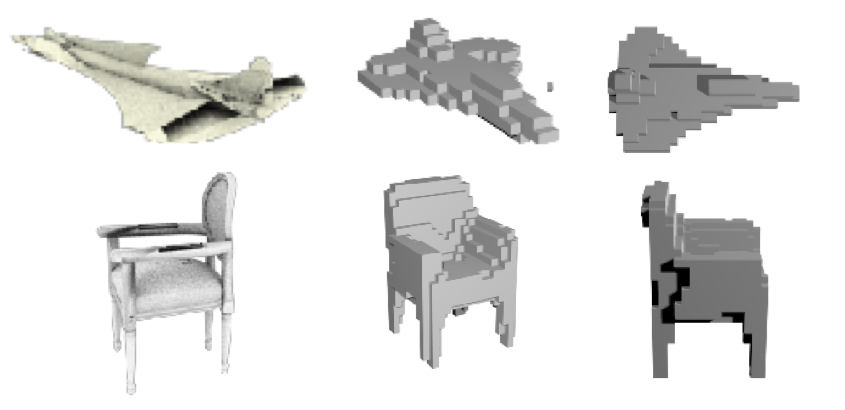
Sample results on ShapeNet dataset using multiple depth images as supervision for training. a) Input image. b,c) Predicted 3D shape.
|
|
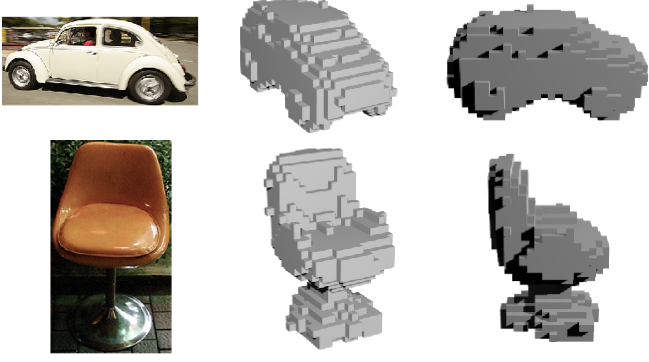
Sample results on PASCAL VOC dataset using pose and foreground masks as supervision for training. a) Input image. b,c) Predicted 3D shape.
|
Driving Sequences as Multi-view Supervision (Cityscapes)
|
|
Multi-view Color Images as Supervision (ShapeNet)
|
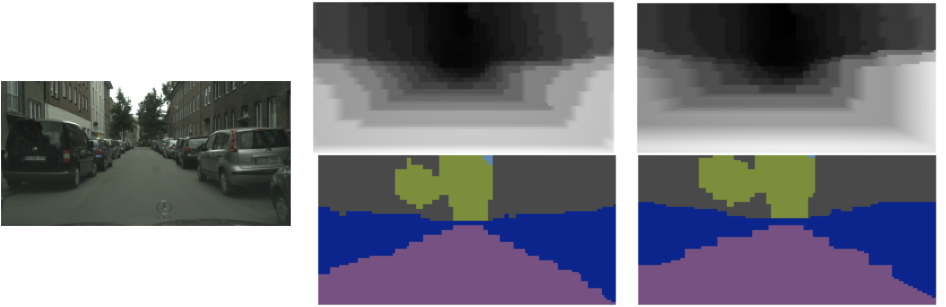
Sample results on Cityscapes dataset using multiple depth and semantic segmentation images seen in driving sequences as supervision for training. a) Input image. b,c) Predicted 3D shape visualized by rendering inferred depth and semantics under simulated forward motion.
|
|
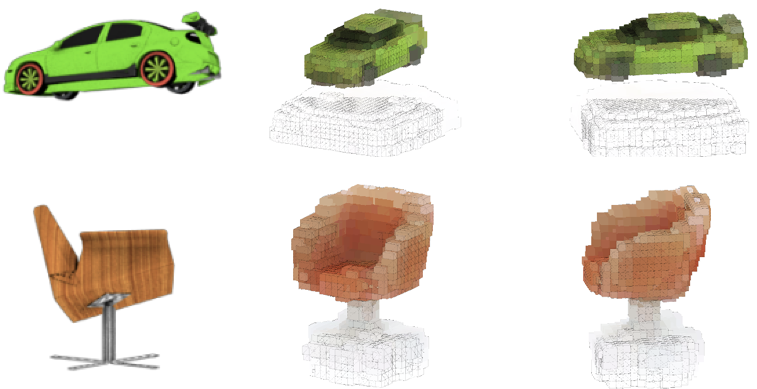
Sample results on ShapeNet dataset using multiple RGB images as supervision for training. a) Input image. b,c) Predicted 3D shape.
|